Important paper from Google on large batch optimization. They do impressively careful experiments measuring # iterations needed to achieve target validation error at various batch sizes. The main "surprise" is the lack of surprises. [thread]
https://t.co/7QIx5CFdfJ
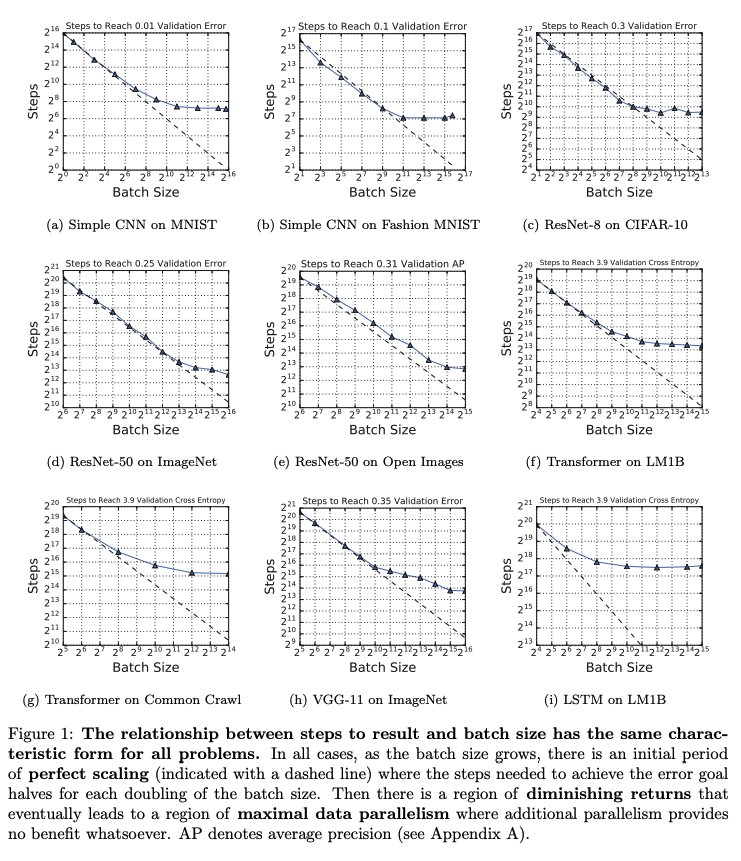
More from Machine learning
10 machine learning YouTube videos.
On libraries, algorithms, and tools.
(If you want to start with machine learning, having a comprehensive set of hands-on tutorials you can always refer to is fundamental.)
🧵👇
1⃣ Notebooks are a fantastic way to code, experiment, and communicate your results.
Take a look at @CoreyMSchafer's fantastic 30-minute tutorial on Jupyter Notebooks.
https://t.co/HqE9yt8TkB
2⃣ The Pandas library is the gold-standard to manipulate structured data.
Check out @joejamesusa's "Pandas Tutorial. Intro to DataFrames."
https://t.co/aOLh0dcGF5
3⃣ Data visualization is key for anyone practicing machine learning.
Check out @blondiebytes's "Learn Matplotlib in 6 minutes" tutorial.
https://t.co/QxjsODI1HB
4⃣ Another trendy data visualization library is Seaborn.
@NewThinkTank put together "Seaborn Tutorial 2020," which I highly recommend.
https://t.co/eAU5NBucbm
On libraries, algorithms, and tools.
(If you want to start with machine learning, having a comprehensive set of hands-on tutorials you can always refer to is fundamental.)
🧵👇
1⃣ Notebooks are a fantastic way to code, experiment, and communicate your results.
Take a look at @CoreyMSchafer's fantastic 30-minute tutorial on Jupyter Notebooks.
https://t.co/HqE9yt8TkB
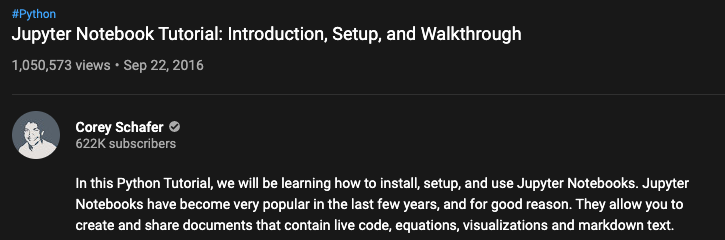
2⃣ The Pandas library is the gold-standard to manipulate structured data.
Check out @joejamesusa's "Pandas Tutorial. Intro to DataFrames."
https://t.co/aOLh0dcGF5
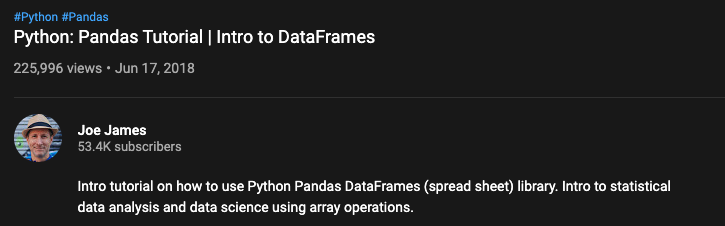
3⃣ Data visualization is key for anyone practicing machine learning.
Check out @blondiebytes's "Learn Matplotlib in 6 minutes" tutorial.
https://t.co/QxjsODI1HB
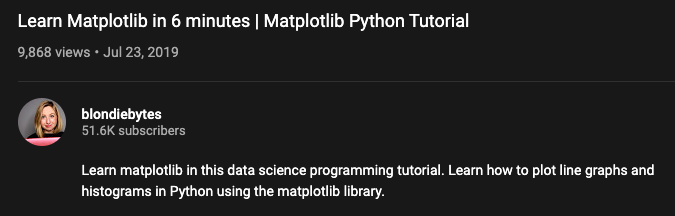
4⃣ Another trendy data visualization library is Seaborn.
@NewThinkTank put together "Seaborn Tutorial 2020," which I highly recommend.
https://t.co/eAU5NBucbm
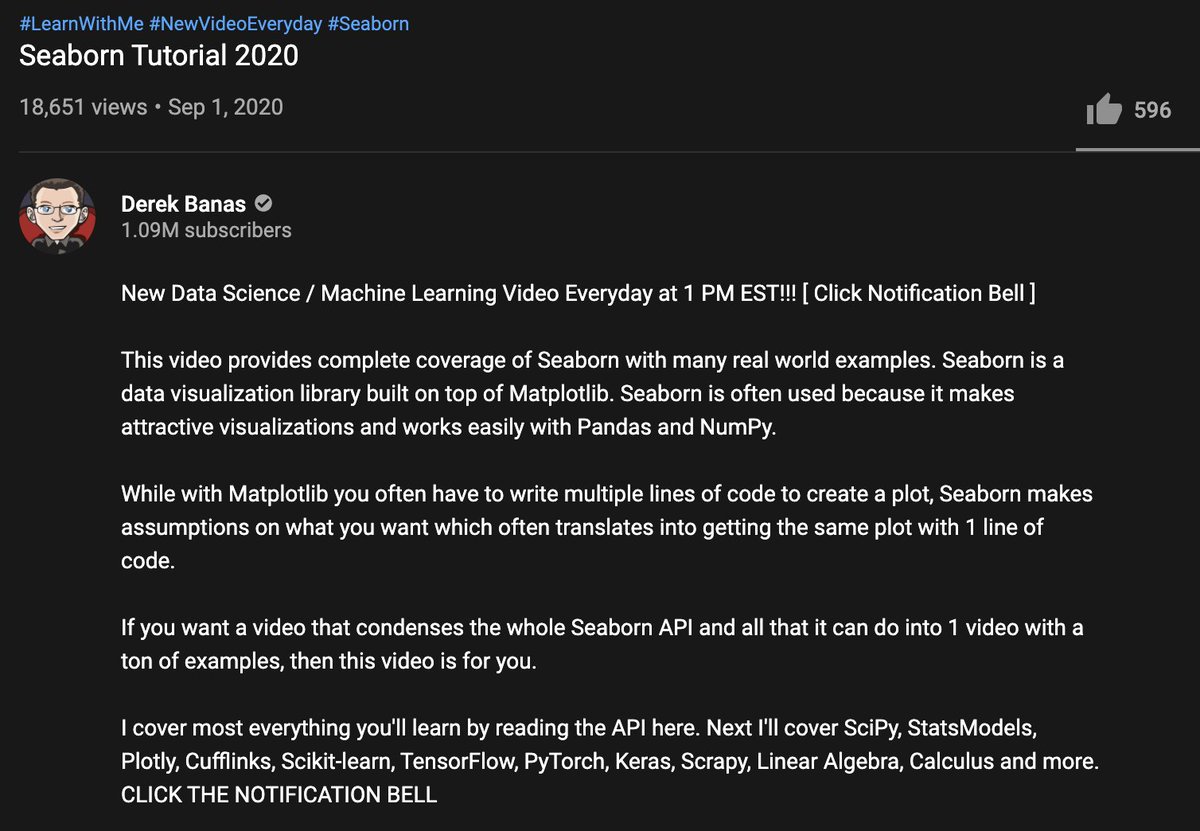