You wouldn't use a jackhammer to nail a painting to the wall.
Machine translation can be a wonderful translation tool, but its uses are widely misunderstood.
Let's talk about Google Translate, its current state in the professional translation industry, and why robots are terrible at interpreting culture and context.
You wouldn't use a jackhammer to nail a painting to the wall.
Certain language pairs are better suited for MT. Typically, the more similar the grammar structure, the better the MT will be. Think Spanish <> Portuguese vs. Spanish <> Japanese.
https://t.co/yiVPmHnjKv

Poor applications of MTPE make human translators miserable--and likely, your clients, too.
(You thought you were going to get out of it this time? Who do you think I am?)
Same word. Different sociocultural context.
But what happens when you take that fish out of the tank and plop it into a completely different one?
Unfortunately, culture is hard to change, so we make these changes to the fish itself for it to thrive in its new environment.
It's not just for translation, too--moving a fish from an British tank to an American tank requires localization, too. ("What the hell is a car 'bonnet'?")
Many contextual systems--a polysystem. Polysystem theory!
And yet.
Despite memeing on MT all the time.
Sometimes, sadly, it's capitalism. Sure, it's not good, but if it'll get a few more people to buy it, who cares?
Not only are you paying for the cost of translation, you're also paying designers for graphics (see: P5R!), additional QA to ensure the translations display correctly, and additional marketing reps in other languages.

First and foremost, we've got to inform developers and producers in the industry of the value of good localization--and why human translators are the best way to ensure your loc is good.
More from Tech
Here is a simple example of a machine learning model.
I put it together a long time ago, and it was very helpful! I sliced it apart a thousand times until things started to make sense.
It's TensorFlow and Keras.
If you are starting out, this may be a good puzzle to solve.
The goal of this model is to learn to multiply one-digit
I put it together a long time ago, and it was very helpful! I sliced it apart a thousand times until things started to make sense.
It's TensorFlow and Keras.
If you are starting out, this may be a good puzzle to solve.
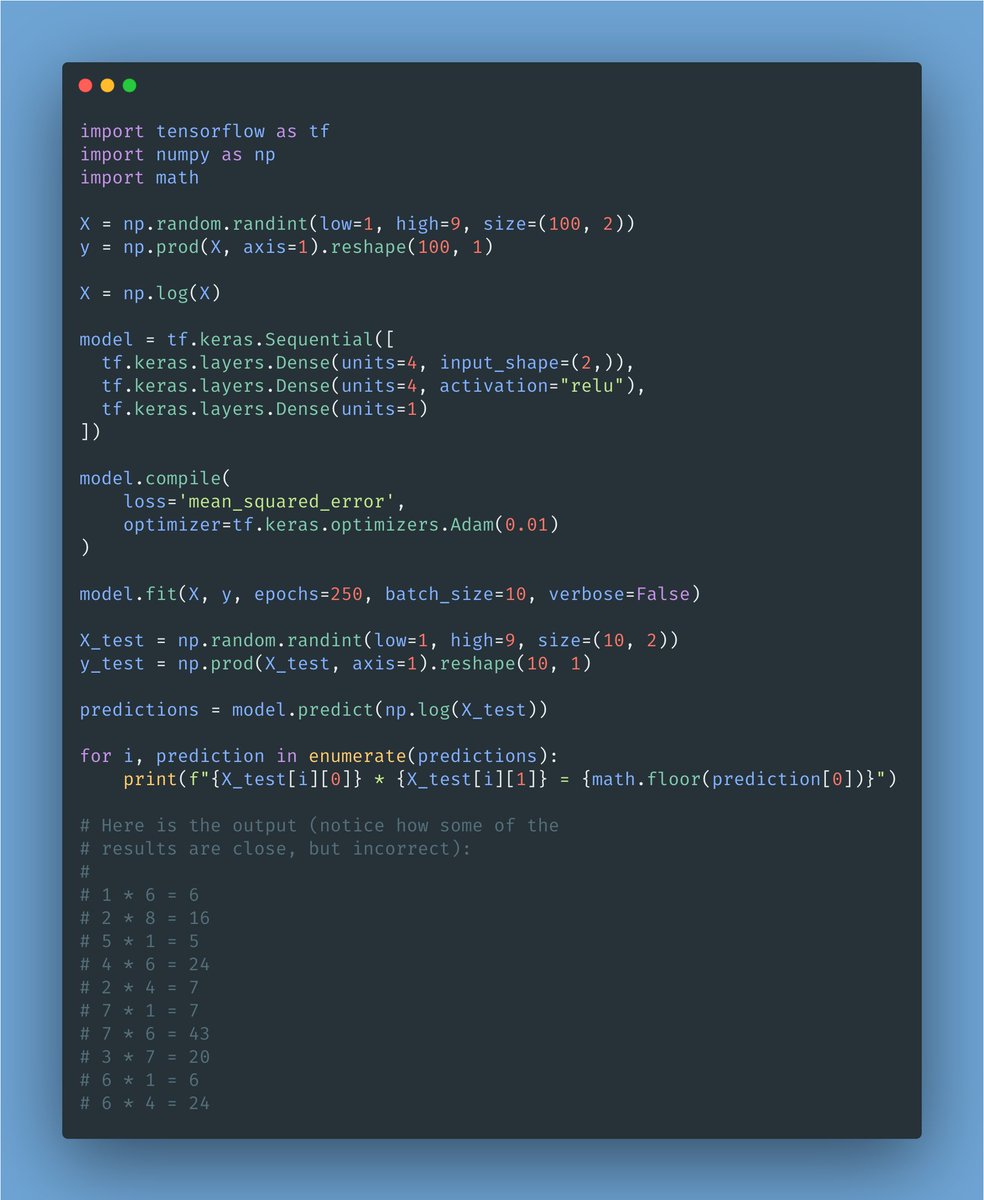
The goal of this model is to learn to multiply one-digit
It is a good example of coding, what is the model?
— Freddy Rojas Cama (@freddyrojascama) February 1, 2021
You May Also Like
Joshua Hawley, Missouri's Junior Senator, is an autocrat in waiting.
His arrogance and ambition prohibit any allegiance to morality or character.
Thus far, his plan to seize the presidency has fallen into place.
An explanation in photographs.
🧵
Joshua grew up in the next town over from mine, in Lexington, Missouri. A a teenager he wrote a column for the local paper, where he perfected his political condescension.
2/
By the time he reached high-school, however, he attended an elite private high-school 60 miles away in Kansas City.
This is a piece of his history he works to erase as he builds up his counterfeit image as a rural farm boy from a small town who grew up farming.
3/
After graduating from Rockhurst High School, he attended Stanford University where he wrote for the Stanford Review--a libertarian publication founded by Peter Thiel..
4/
(Full Link: https://t.co/zixs1HazLk)
Hawley's writing during his early 20s reveals that he wished for the curriculum at Stanford and other "liberal institutions" to change and to incorporate more conservative moral values.
This led him to create the "Freedom Forum."
5/
His arrogance and ambition prohibit any allegiance to morality or character.
Thus far, his plan to seize the presidency has fallen into place.
An explanation in photographs.
🧵
Joshua grew up in the next town over from mine, in Lexington, Missouri. A a teenager he wrote a column for the local paper, where he perfected his political condescension.
2/
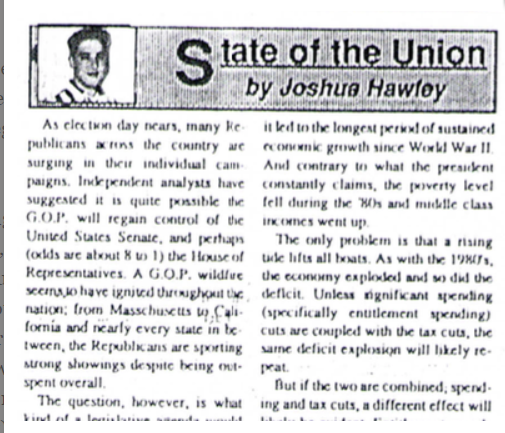
By the time he reached high-school, however, he attended an elite private high-school 60 miles away in Kansas City.
This is a piece of his history he works to erase as he builds up his counterfeit image as a rural farm boy from a small town who grew up farming.
3/
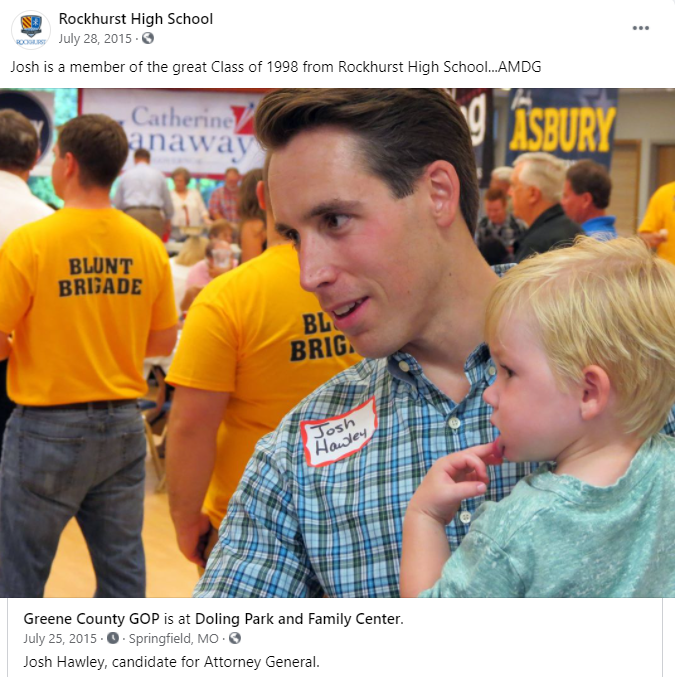
After graduating from Rockhurst High School, he attended Stanford University where he wrote for the Stanford Review--a libertarian publication founded by Peter Thiel..
4/
(Full Link: https://t.co/zixs1HazLk)
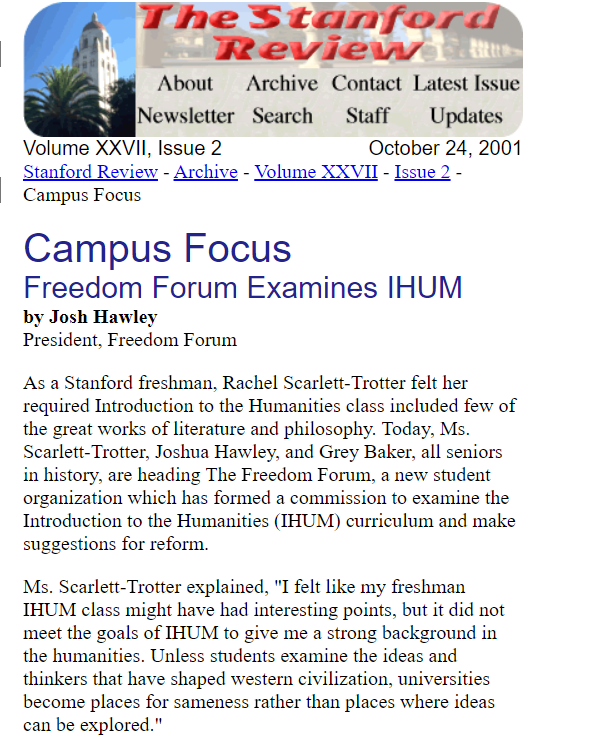
Hawley's writing during his early 20s reveals that he wished for the curriculum at Stanford and other "liberal institutions" to change and to incorporate more conservative moral values.
This led him to create the "Freedom Forum."
5/
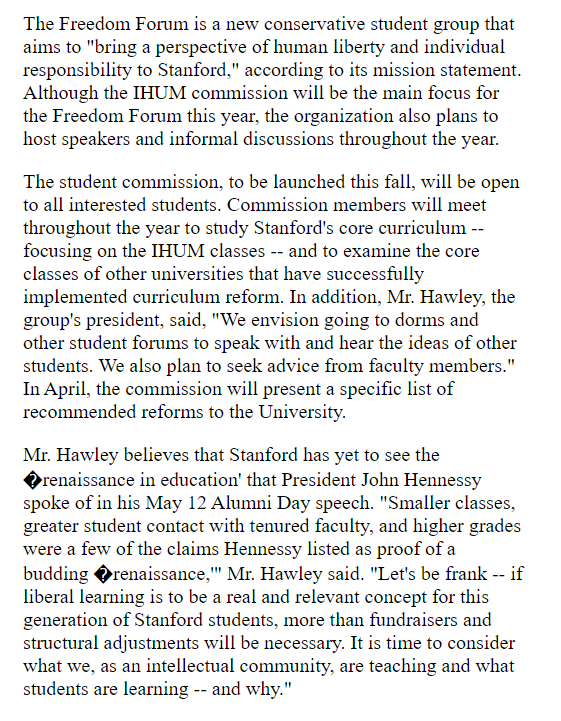