1/🧵 Good #DataScience advice that breaks pretty much every rule you learned in class... a thread. (+full blog post linked)
English version: https://t.co/dG4l6vPFBT
Spanish version: https://t.co/gFAjPQ5clS
#AI #MachineLearning #Statistics #RStats
For more info: https://t.co/Ue332SMjy1
More from Machine learning
Really enjoyed digging into recent innovations in the football analytics industry.
>10 hours of interviews for this w/ a dozen or so of top firms in the game. Really grateful to everyone who gave up time & insights, even those that didnt make final cut 🙇♂️ https://t.co/9YOSrl8TdN
For avoidance of doubt, leading tracking analytics firms are now well beyond voronoi diagrams, using more granular measures to assess control and value of space.
This @JaviOnData & @LukeBornn paper from 2018 referenced in the piece demonstrates one method https://t.co/Hx8XTUMpJ5
Bit of this that I nerded out on the most is "ghosting" — technique used by @counterattack9 & co @stats_insights, among others.
Deep learning models predict how specific players — operating w/in specific setups — will move & execute actions. A paper here: https://t.co/9qrKvJ70EN
So many use-cases:
1/ Quickly & automatically spot situations where opponent's defence is abnormally vulnerable. Drill those to death in training.
2/ Swap target player B in for current player A, and simulate. How does target player strengthen/weaken team? In specific situations?
>10 hours of interviews for this w/ a dozen or so of top firms in the game. Really grateful to everyone who gave up time & insights, even those that didnt make final cut 🙇♂️ https://t.co/9YOSrl8TdN
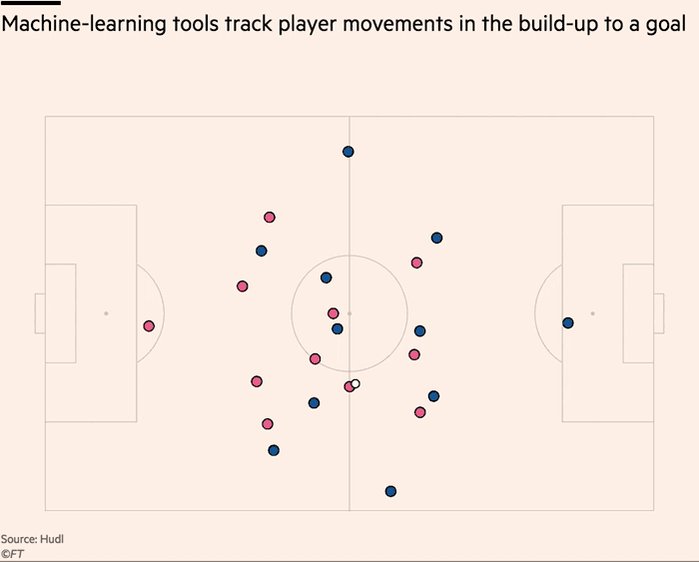
For avoidance of doubt, leading tracking analytics firms are now well beyond voronoi diagrams, using more granular measures to assess control and value of space.
This @JaviOnData & @LukeBornn paper from 2018 referenced in the piece demonstrates one method https://t.co/Hx8XTUMpJ5
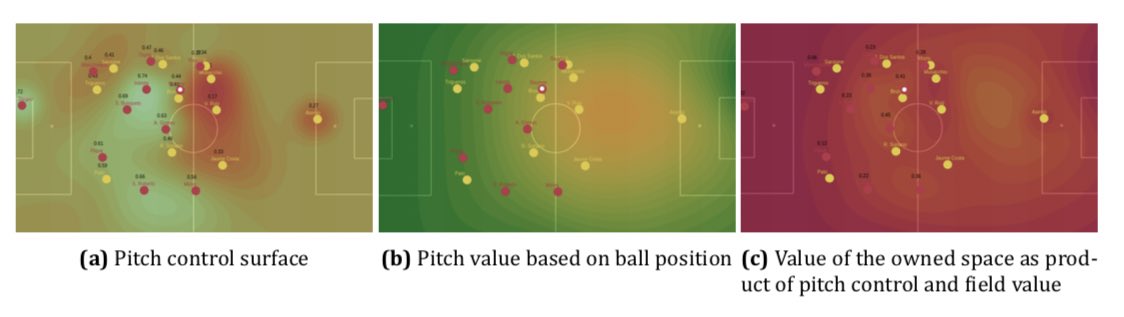
Bit of this that I nerded out on the most is "ghosting" — technique used by @counterattack9 & co @stats_insights, among others.
Deep learning models predict how specific players — operating w/in specific setups — will move & execute actions. A paper here: https://t.co/9qrKvJ70EN
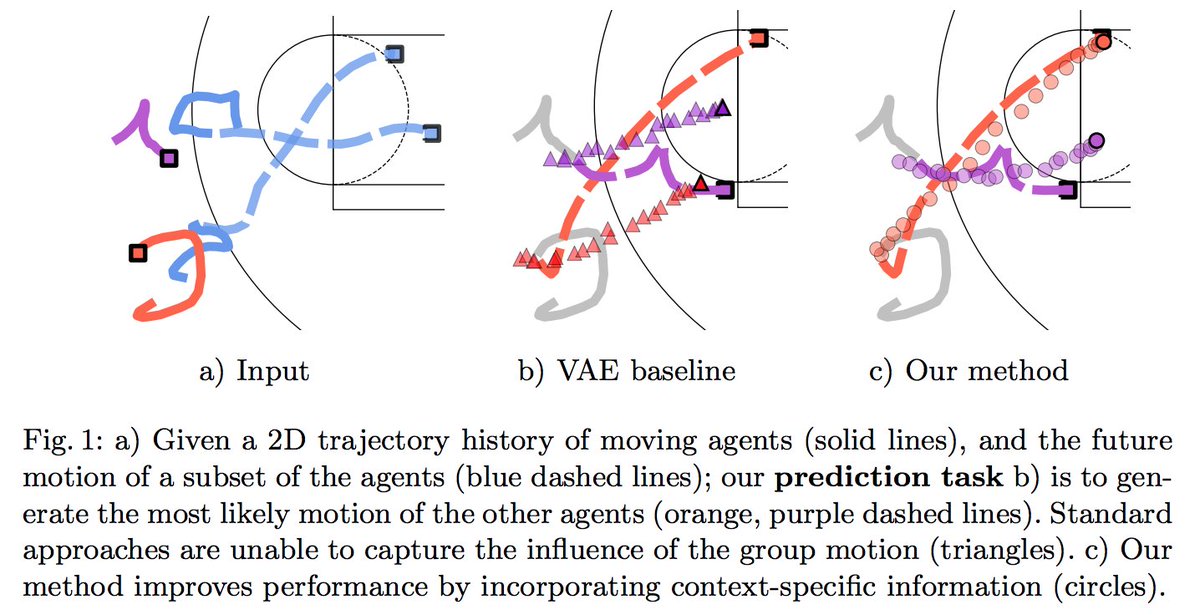
So many use-cases:
1/ Quickly & automatically spot situations where opponent's defence is abnormally vulnerable. Drill those to death in training.
2/ Swap target player B in for current player A, and simulate. How does target player strengthen/weaken team? In specific situations?
Do you want to learn the maths for machine learning but don't know where to start?
This thread is for you.
🧵👇
The guide that you will see below is based on resources that I came across, and some of my experiences over the past 2 years or so.
I use these resources and they will (hopefully) help you in understanding the theoretical aspects of machine learning very well.
Before diving into maths, I suggest first having solid programming skills in Python.
Read this thread for more
These are topics of math you'll have to focus on for machine learning👇
- Trigonometry & Algebra
These are the main pre-requisites for other topics on this list.
(There are other pre-requites but these are the most common)
- Linear Algebra
To manipulate and represent data.
- Calculus
To train and optimize your machine learning model, this is very important.
This thread is for you.
🧵👇
The guide that you will see below is based on resources that I came across, and some of my experiences over the past 2 years or so.
I use these resources and they will (hopefully) help you in understanding the theoretical aspects of machine learning very well.
Before diving into maths, I suggest first having solid programming skills in Python.
Read this thread for more
Are you planning to learn Python for machine learning this year?
— Pratham Prasoon (@PrasoonPratham) February 13, 2021
Here's everything you need to get started.
\U0001f9f5\U0001f447
These are topics of math you'll have to focus on for machine learning👇
- Trigonometry & Algebra
These are the main pre-requisites for other topics on this list.
(There are other pre-requites but these are the most common)
- Linear Algebra
To manipulate and represent data.
- Calculus
To train and optimize your machine learning model, this is very important.