If someone doesn’t want to read everything in the full style guide, they can at least come to this section and get the gist.
The eight key components of a social media style guide
A thread 👇🏻👇🏾👇 (with a free template at the end)
If someone doesn’t want to read everything in the full style guide, they can at least come to this section and get the gist.
You have the same voice all the time, but your tone changes. Your voice and tone humanize your brand and let you take part in conversations naturally.
You’ll want to carry over many of your spelling and grammar guidelines from your overall content guide, but keep in mind you may want to modify for social media constraints.
With so many different platforms, formatting on social media is especially important to ensure you are consistent and make your brand recognizable.
You’d be hard pressed to find anything that can inject as much fun and personality into your social media as emoji! (Though it might not be suitable for every platform.)
Hashtags are important for everything from campaigns to joining in conversations. You can include a list of branded and campaign specific hashtags.
Your multimedia usage guidelines can include the content, context, and style (informational, whimsical, etc.).
In today’s increasingly connected world, it’s imperative that your brand be mindful of how you’re perceived on social media, particularly in relation to breaking news stories.
Free template: https://t.co/OWrJ1akQTF
More from All
How can we use language supervision to learn better visual representations for robotics?
Introducing Voltron: Language-Driven Representation Learning for Robotics!
Paper: https://t.co/gIsRPtSjKz
Models: https://t.co/NOB3cpATYG
Evaluation: https://t.co/aOzQu95J8z
🧵👇(1 / 12)
Videos of humans performing everyday tasks (Something-Something-v2, Ego4D) offer a rich and diverse resource for learning representations for robotic manipulation.
Yet, an underused part of these datasets are the rich, natural language annotations accompanying each video. (2/12)
The Voltron framework offers a simple way to use language supervision to shape representation learning, building off of prior work in representations for robotics like MVP (https://t.co/Pb0mk9hb4i) and R3M (https://t.co/o2Fkc3fP0e).
The secret is *balance* (3/12)
Starting with a masked autoencoder over frames from these video clips, make a choice:
1) Condition on language and improve our ability to reconstruct the scene.
2) Generate language given the visual representation and improve our ability to describe what's happening. (4/12)
By trading off *conditioning* and *generation* we show that we can learn 1) better representations than prior methods, and 2) explicitly shape the balance of low and high-level features captured.
Why is the ability to shape this balance important? (5/12)
Introducing Voltron: Language-Driven Representation Learning for Robotics!
Paper: https://t.co/gIsRPtSjKz
Models: https://t.co/NOB3cpATYG
Evaluation: https://t.co/aOzQu95J8z
🧵👇(1 / 12)
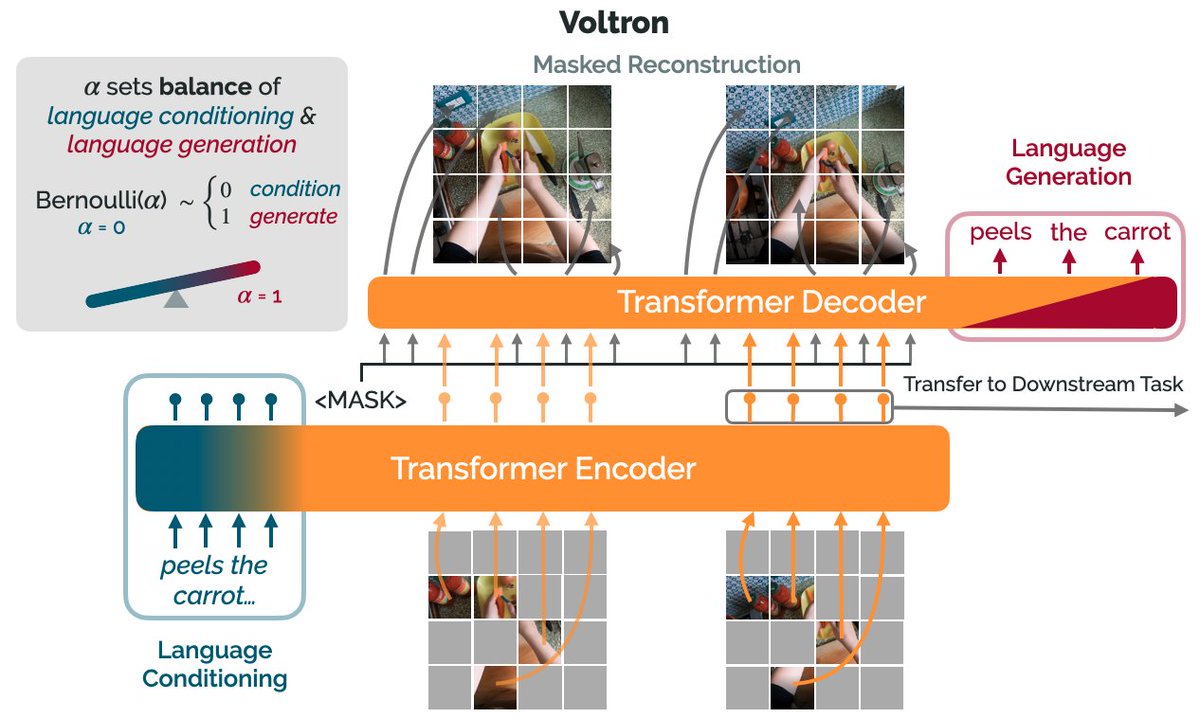
Videos of humans performing everyday tasks (Something-Something-v2, Ego4D) offer a rich and diverse resource for learning representations for robotic manipulation.
Yet, an underused part of these datasets are the rich, natural language annotations accompanying each video. (2/12)
The Voltron framework offers a simple way to use language supervision to shape representation learning, building off of prior work in representations for robotics like MVP (https://t.co/Pb0mk9hb4i) and R3M (https://t.co/o2Fkc3fP0e).
The secret is *balance* (3/12)
Starting with a masked autoencoder over frames from these video clips, make a choice:
1) Condition on language and improve our ability to reconstruct the scene.
2) Generate language given the visual representation and improve our ability to describe what's happening. (4/12)
By trading off *conditioning* and *generation* we show that we can learn 1) better representations than prior methods, and 2) explicitly shape the balance of low and high-level features captured.
Why is the ability to shape this balance important? (5/12)